2025-05-05 11:37
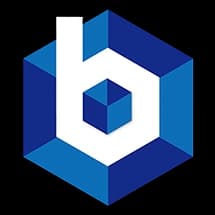
BLOCKMEDIA

Image source: Block Media
# The Challenge of Black Box Large Language Models and Accountability
Large Language Models (LLMs), such as ChatGPT, have revolutionized artificial intelligence by generating human-like text. However, their internal mechanisms remain largely opaque, earning the term "black boxes." Even developers find it challenging to explain these models' decision-making processes. With billions of parameters, LLMs learn autonomously and develop unique decision-making paths, diverging from traditional programming and making it difficult to pinpoint the source of specific outputs.
Experts often cite this opacity as a barrier to trust. Unite.AI notes that advanced LLMs, including GPT-4, operate in ways that are mostly untraceable, making it hard to control biases, errors, or unintended consequences. This lack of transparency raises significant concerns about reliability and safety.
# The Potential to Steer AI: Design Intent and Data Influence
Modern AI can be directed according to developer intentions through mechanisms like training data selection, fine-tuning, system prompts, and human feedback reinforcement learning. Companies such as OpenAI train models to avoid dangerous or explicit content by embedding internal policies into AI decision-making processes. The GPT-4 technical report on arXiv outlines methods, including human feedback and custom system instructions, to achieve these adjustments.
However, such mechanisms also allow external influences. Strategic prompts can guide or bias AI responses, as research on prompt injection published on arXiv has shown. User inputs can manipulate a model's output, sometimes overriding internal system guidelines to align with manipulator intentions.
While these adjustments highlight AI flexibility, they pose risks. External observers cannot easily discern a designer's intentions or how they were implemented in the model, contributing to the black-box paradigm risks.
Uncontrolled or undisclosed biases in AI models can lead to unintended consequences for millions. Backdoor attacks, where specific triggers cause undesirable or hazardous outputs, are an emerging threat. Experiments since 2023 on GPT-series models and open-source counterparts have shown that hidden triggers can manipulate models toward predefined outputs.
Moreover, AI models risk absorbing harmful or biased information from online content, resulting in discriminatory or erroneous outputs. Instances of AI bias against demographics or providing incorrect medical information underscore this issue. The black-box nature of LLMs makes it difficult to identify if such failures stem from training data, structural flaws, or tuning processes, posing a significant challenge for researchers.
# The Imperative of Interpretability in AI Development
Addressing these risks demands progress in mechanistic interpretability, which involves tracing neurons and circuits in AI systems to analyze concept structuring and decision-making influences. This is akin to mapping the human brain and identifying which elements contribute to specific results.
Research by Anthropic on its Claude model identified millions of internal concepts and demonstrated the ability to manipulate them, altering the model’s behavior. This breakthrough serves as an early tool for analyzing AI "thought" processes.
Enhanced interpretability enables several key capabilities:
- Early detection of hidden biases, errors, or risk signals within models,
- Identification of potential backdoors or trigger conditions, and
- Visualization of decision pathways for improved transparency, aiding stakeholders in mitigating risks effectively.
One study described interpretability technologies as "cornerstones for AI safety alignment" and essential for ensuring legal and societal accountability in AI.
# Moving Forward: Emphasizing Transparency, Interpretability, and Accountability
As AI becomes increasingly powerful, the need to interpret and regulate its operations becomes critical. While technological advancements progress rapidly, interpretability and governance have lagged—a gap requiring urgent attention to avoid irreversible consequences.
Risk mitigation policies should prioritize:
- Continuous investment in interpretability research,
- Transparency and disclosure by AI companies and research institutions,
- Structural responses like retraining when issues are identified, rather than abandoning models,
- Developing and legislating frameworks for detecting AI biases and risks.
AI has evolved from a mere tool to an autonomous, complex system shaped by human intent and potentially unintended consequences. Humanity has a duty to establish clear standards, guide AI's development, and maintain rigorous oversight.
Without decisive action, AI systems might operate beyond human control, leading to unpredictable and possibly harmful outcomes. However, proactive measures today can ensure this technology benefits society and future generations.
View original content to download multimedia: https://www.blockmedia.co.kr/archives/900755